367. CubeSate Cluster Deployment Tracking
Team
Name | Role | Primary |
Penina Axelrad | Principal Investigator | ● |
David Klaus | Principal Investigator | |
Stephen Earle | Tech Monitor | ● |
Jennifer Huettl | Fiscal Admin | ● |
Stephanie Rosario | Fiscal Admin | ● |
Andy Wang | Fiscal Admin | ● |
Research Area
1.0 Space Traffic Management & Spaceport Operations
Project Description
Clustered CubeSat deployments, where dozens of CubeSats are released over a short time span, represent a relatively new and challenging problem for detection, tracking, and space traffic management. Over the last few years CubeSat missions have reported on the difficulty of relying on Two Line Elements (TLEs) provided by the Joint Space Operations Center (JSpOC) early in the mission. Researchers and industry professionals have begun taking an interest in this scenario. Mainly they are advocating policy changes to push CubeSat developers toward adding navigation aids, in the form of ID beacons or reflectors. We propose to develop a robust solution that leverages, but is not entirely reliant on compliance by CubeSat developers. Our goal is to develop and demonstrate a resilient strategy for the deployment, detection, and tracking of multiple CubeSats. The proposed research for the first year focuses on solving the estimation problem of detecting, tracking and identifying each individual CubeSat in a realistic large scale deployment scenario. Multi-‐target estimation methods based on random finite set (RFS) statistics, including the cardinalized probability hypothesis density (CPHD) and the labelled multi-‐Bernoulli (LMB) filter provide the basis for this work. Using the understanding gained from the first year study, the second year will focus on recommending deployment strategies and accelerating the estimation process, using reported data from a subset of cooperative, fully functioning CubeSats in the deployment. The result will be a validated set of algorithms to support and enhance the safety and speed at which future CubeSat deployments can achieve their mission goals. Funding is requested at the level of $75K per year to support PhD student, John Gaebler, who is expected to graduate in May 2019, with this project forming the primary basis for his doctoral dissertation.
Project Outcomes
The proposed research benefits space traffic management of CubeSat deployments as follows:
• Improved tracking and accurate identification allows satellite operators to reliably access their spacecraft, insuring that time critical commands are issued and system data downloaded in time to address any possible issues.
• Reducing the time to establish orbits/TLE’s for CubeSats allows operators to more quickly begin their operational mission. In LEO, where lifetimes can be measured in months, additional weeks and days contribute meaningfully to overall utility.
• Better orbit predictions, especially after closely spaced deployments, allow for more informed conjunction assessments.
The approaches may also have potential applicability to debris tracking, especially for debris resulting from a break-‐up event.
Summary of Output
Space traffic surveillance and management requires timely, cost effective, and robust approaches to accurately tracking, tagging, and predicting the orbits of large groups of CubeSat class satellites. Thus, the goal of this project is to develop optimal deployment and tracking approaches for large clusters of CubeSats to achieve the following objectives:
• Maximize likelihood of nominal deployment of all CubeSats (minimize risk of collisions).
• Minimize time to correctly identify each CubeSat.
• Maximize the accuracy of the orbit knowledge of each deployed CubeSat.
• Accurately predict impending collisions with longest possible lead time.
Quad Chart
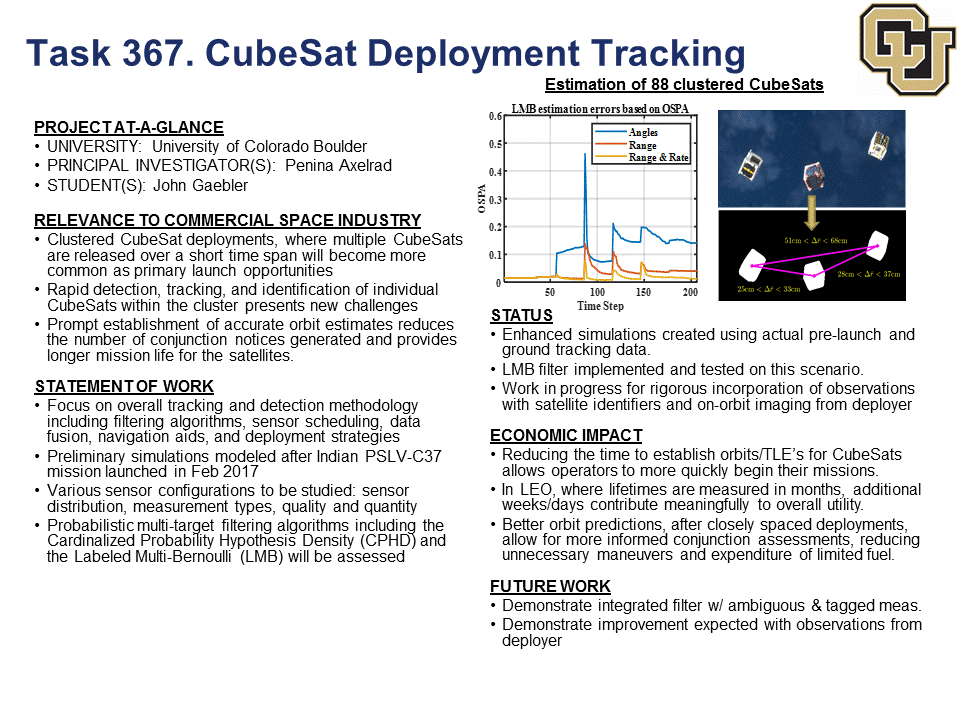